Helping KP Washington lead in intelligent health care
How our Learning Health System Program is using statistical methods and machine learning techniques to respond to COVID-19
Since the COVID-19 pandemic hit, predictive modeling has been helping guide the critical decisions that national, state, and local leaders need to make in the face of uncertainty. Predictive modeling can also help health systems decide how best to deploy limited resources, especially during times of crisis. We talked with Emily Westbrook, MHA, about how Kaiser Permanente Washington’s Learning Health System (LHS) Program has been helping the organization develop and deploy predictive risk models—both before and since COVID-19—and what work is on the horizon. Emily is the Director of Research Operations and Programs at Kaiser Permanente Washington Health Research Institute (KPWHRI) and the operations lead for the LHS Program.
Can you tell us briefly how predictive modeling works and give an example of its use in health care?
Predictive modeling uses data analysis, machine learning, and statistics to identify patterns in data and recognize the chance of specific events occurring. One way predictive modeling is used in health care is to understand who in a population might be at risk of a harmful event or a negative health outcome. For example, a predictive model can help identify individuals with high medical need or disease burden who are at high risk of being hospitalized in the next 12 months.
How has the Learning Health System Program been involved in developing predictive models at Kaiser Permanente Washington—both before and after COVID-19?
Over the last several years, the Learning Health System Program has been part of an emerging interdisciplinary partnership to develop methods for responsible implementation of risk prediction models in care delivery at Kaiser Permanente Washington. This work brings together expertise from the Research Institute, Care Delivery, Care Delivery Technology Services, Finance, Business Intelligence, and other areas of the organization.
Our key successes before the COVID-19 pandemic were to develop, validate, and deploy risk prediction models that have:
- Increased flu vaccination rates among members at risk of hospitalization (see story from KING5 News)
- Introduced additional mental health screening for members at high risk of suicide attempt
- Reduced appointment no shows and late cancellations with additional text message reminders
Additionally, we’ve been exploring other uses of risk prediction models to help identify members who could benefit from palliative care services or members who could benefit from additional case management because they are at higher risk for a prolonged hospital stay.
When COVID-19 hit, we were able to utilize existing infrastructure from our flu vaccination intervention to rapidly deploy outreach efforts to Medicare members to make sure their care needs were being addressed. We were able to then quickly expand this to include outreach efforts to non-Medicare members with complex medical and social needs and other chronic conditions.
Can you say more about how having an interdisciplinary team is important for responsibly using predictive models?
Having an interdisciplinary team is critical because it helps us avoid pitfalls that can lead to biased models. One example in the press recently is a health care risk-prediction algorithm that exacerbates health inequities.
The reason for the bias in this model is that it uses health care spending as a proxy for health care needs. But we know that structural racism impacts access, treatment, and outcomes for Black, Indigenous, and People of Color, and less money is spent on Black patients than is actually required to meet their needs. Thus, using a model based on health care spending channels a beneficial resource to white patients and withholds it from Black patients who have higher needs.
Risk models also have other important limitations that we need to be aware of. For example, models aren’t helpful unless they are matched with an evidence-based intervention that is correctly balanced with the accuracy of the model. If the intervention is low-risk and low-cost to the health system, it is not as critical to have a model with perfect accuracy to predict the outcome. If the intervention is potentially high-risk and high-cost to the organization, it would be important to have a model with very high accuracy to predict the outcome.
KPWHRI Assistant Investigator Yates Coley, PhD, has been instrumental in investigating and addressing racial and other types of bias in the clinical prediction algorithms we develop. A biostatistician and CATALyST K12 Learning Health System scholar, Yates is evaluating the performance of our suicide attempt risk prediction model across racial and ethnic subgroups to ensure that all patients benefit from the use of predictive analytics. Matching sophisticated statistical techniques with expert interdisciplinary teams helps us ensure the models we develop and deploy are accurate, actionable, and equitable.
What’s next for your team as our health system and our members continue to adapt to COVID-19?
As the pandemic unfolded, anecdotal evidence emerged suggesting that there could be significant decreases in the use of acute care during COVID-19 because people are afraid of contracting the virus—so afraid that they may not be seeking care when they really need it. Again, this is where the Learning Health System Program can help: We can use expert analytic methods to understand the extent of the problem and to identify ways to intervene and help prevent negative health outcomes for our members.
Our team is now focused on some key next steps, including:
- Analyzing patterns of acute care utilization at Kaiser Permanente Washington since the onset of COVID-19 to understand if there’s been a similar reduction in care as observed in two recent peer-reviewed studies—one published in JAMA and the other in the New England Journal of Medicine.
- Looking for characteristics that might help us understand patterns of delaying care due to fear of contracting COVID-19 during the height of the pandemic. This could help inform future targeted interventions to prevent delays in care in the future.
- Continuing to build on existing predictive analytic work to identify members who would benefit from additional routine, proactive outreach to ensure all care needs are being met.
Our ultimate goal is to harness the full power of being an integrated health system that combines care and coverage and has robust data sources to ensure that we are delivering on the promise of being the best place to receive care. We are in a unique position to really use the data we have wisely to provide high-quality, personalized care to our members during a time when they need it most.
Related news
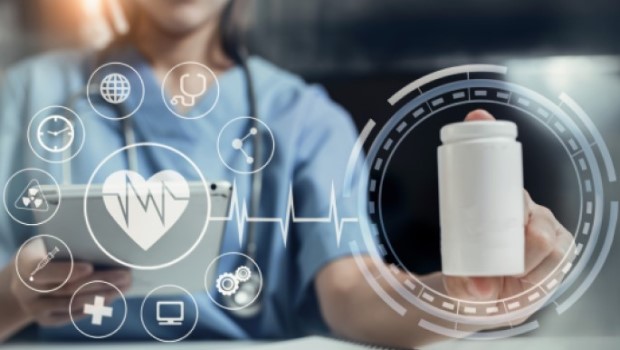
Risk model uses advanced analytics to guide informed treatment decisions at KP Washington
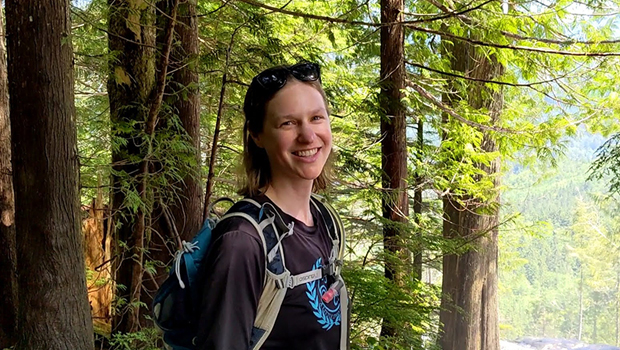